RNAi Technology Arrives
The year is 2006, and Andrew Fire and Craig Mello are about to receive the Nobel Prize in Physiology or Medicine for the joint discovery of RNA interference (RNAi), a method for turning off or “silencing” genes using RNA molecules. In the years leading up to this moment, RNAi technology had positioned itself as a new class of blockbuster medicine that promised to revolutionize the biopharmaceutical landscape. Because RNAi actuates at the gene-expression level, it opened the door for tackling untargetable proteins before they were even made, circumventing drug tolerance mechanisms and opening new avenues for gene therapy. But even with Fire and Mello’s prizewinning innovation, another 12 years of research and trials would pass before scientists and clinicians could bring the first RNAi therapy to patients.
RNAi is a process that turns off specific genes in a cell by using RNA sequences. It was discovered in the 1990s when scientists added double-stranded (ds) RNA encoding to petunia flowers, which they were surprised to find turned the petals white rather than deepening their purple color. They expected these dsRNA sequences to behave like a messenger RNA (mRNA) and carry information to the flowers’ protein synthesis centers and produce purple pigment. But in this case, rather than leading to protein synthesis, information contained in dsRNA turned off the natural pathway for purple pigment expression. These dsRNA molecules are now known as siRNA (small interfering RNA) and are the central agents in RNAi, where they act by binding to homologous mRNA molecules, leading to their degradation and the shutdown of the corresponding gene.
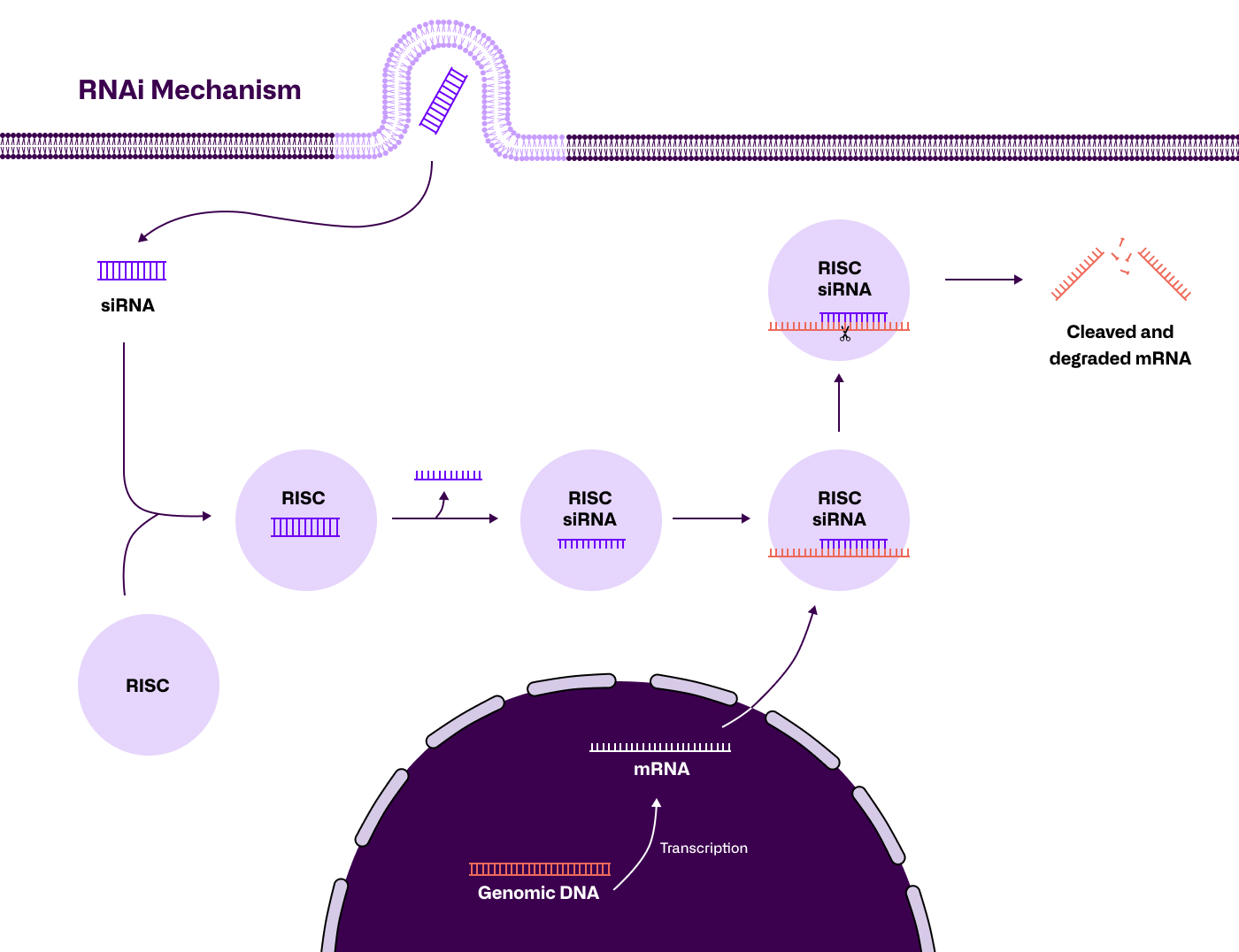
Large pharmaceutical companies invested billions of dollars in RNAi startups, but after a few years and several clinical-stage failures, enthusiasm waned. Several companies, including Roche, Novartis and Pfizer, terminated partnerships and scaled back efforts. Despite comprehending the RNAi mechanism itself, they still needed to determine how to appropriately deliver RNAi and avoid immunogenicity effects before RNAi therapies could truly take off. Breakthroughs in this regard lead to the development and approval of five new RNAi therapies since 2018, providing alternatives to patients with previously untreated rare genetic disorders, such as hereditary transthyretin-mediated amyloidosis (~50,000 individuals worldwide) and primary hyperoxaluria type 1 (~150,000 individuals worldwide).
In 2022, gene expression-inhibition drugs climbed to the sixth-largest mechanism of action in clinical drug discovery pipelines, which suggests that in the future, companies will put more effort into RNAi. However, selecting the most effective siRNA for a given gene is challenging, as there may be thousands of potential siRNAs to choose from. Predicting the inhibition efficiency of siRNAs is therefore important in order to select the most active candidates. This process is a significant problem for researchers working in the field of RNAi and a major expense in the RNAi discovery pipeline.
RNAi Design: Principles and Challenges
For an RNAi therapy to work, first and foremost its specificity and efficacy must be ensured. That is, ensuring the RNAi targets only the specific gene of interest, and that it does so in an effective manner. Other essential aspects include avoiding the activation of the innate immune system, guaranteeing zero toxicity and ensuring a long half-life/slow degradation in the body circulation and inside cells. While a myriad of chemical modifications and delivery mechanisms not covered in this post can achieve the latter, the secrets for specificity and efficacy are enclosed in the RNA sequence itself.
Specificity is achieved by filtering out siRNAs with complete/partial homology with unintended gene transcripts (also including their 3’UTR regions). Some design parameters can also be controlled to help reduce the likelihood of unspecific binding to unwanted sequences, such as GC content and seed sequence thermal stability. In addition, incorporating strand asymmetry into the design process maximizes the loading of the correct strand (i.e. guide strand) of the siRNA duplex into RISC, which also minimizes unwanted potential activity from the opposite strand (i.e. passenger strand).
Efficacy, on the other hand, is harder to predict and control for because it is impacted by several factors, such as resistance to degradation, rate of guide strand loading into RISC, thermodynamics and the target’s mRNA-related features, including accessibility and secondary structure formation. All of these factors and others are directly influenced by the nucleotide composition of both the siRNA duplex and the target’s mRNA. Over the years, researchers compiled rule-based approaches for siRNA sequence composition to select high-potential siRNAs. These rules revolve around placing specific nucleotides at determined positions (e.g. presence of A at positions 4 and 19 of the sense strand) or rules of thumb for mRNA region selection (e.g. 50–100 nucleotides downstream of the start codon). Many of these rules likely reflect the underlying factors implied in siRNA efficacy (thermodynamics, accessibility, etc), but it is also likely that not all of them contribute equally to efficacy and that other more quantitative approaches are better suited for the prediction of siRNA efficacy.
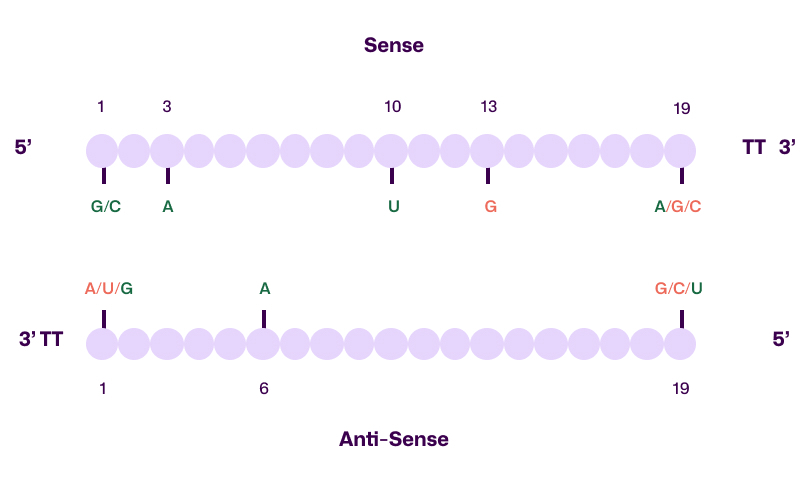
Machine learning methods are a viable alternative to rule-based approaches, as they can better balance the importance of all features of interest, provided that they have sufficient data from which to learn patterns. Luckily, researchers have compiled several datasets (Huesken, Reynolds, Ui-Tei, etc) which allowed the development of ML and DL models that consistently outperform rule-based approaches.
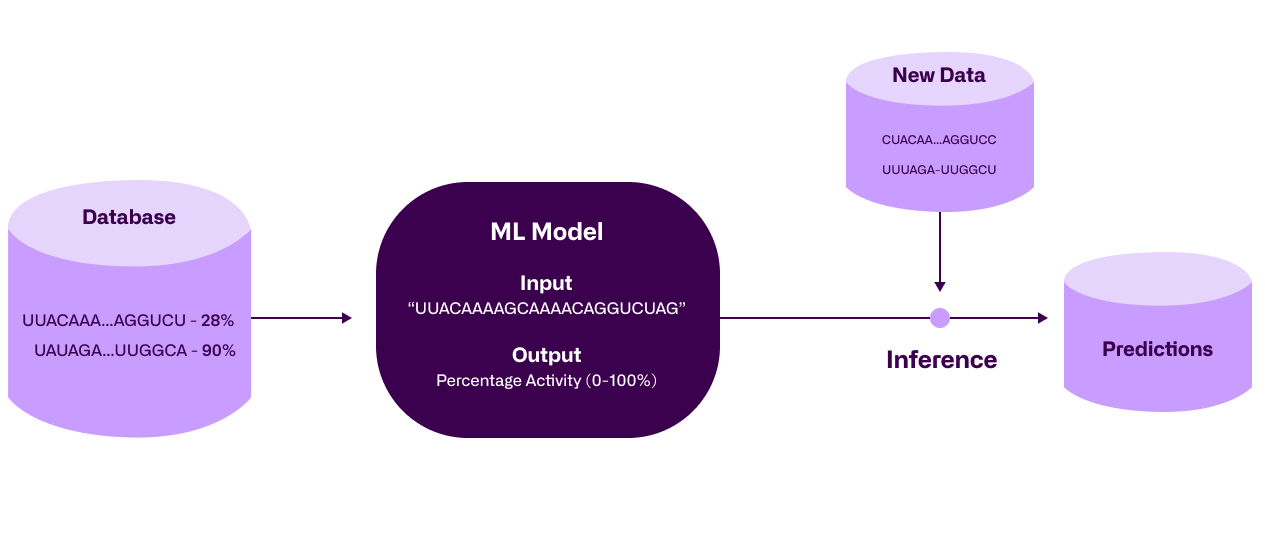
Despite these efficiencies, designing effective siRNA molecules for targeted gene silencing remains a challenge for R&D teams without ML expertise. The main option for in-house siRNA design is using public web apps or open-source repositories. These platforms offer free prediction capabilities using both rule-based and machine learning methods, but they come with limitations. Perhaps the most significant disadvantage is the use of outdated predictive technology. For example, most ML-based approaches use SVMs that have fallen behind in the last decade to more powerful models, specially within the sequence data domain, such as LSTMs and transformers. Ultimately, this outdated technology leads to a lower success rate for generated siRNAs.
Additionally, the methodology employed by these platforms is not tailored to the unique needs of each lab's data and schema, and they lack APIs or integration options with the lab's main data platform, making them ill-suited for use in a production setting. An alternative is to hire bioinformatic consultants or to order siRNAs from third-party manufacturers, but this comes with its own set of challenges related to cost, time and privacy. Besides being an expensive and time-consuming process, requiring weeks to produce results, the methodology used by the consultant lacks transparency, which may fall into the aforementioned pitfalls, and most importantly, may not adhere to privacy standards of organizations conducting clinical drug discovery. These are the limitations and problems that Loka set out to solve.
Introducing Petunia
Loka developed Petunia to answer the most urgent challenges in the RNAi development. Petunia is a customizable machine learning platform that serves as the go-to tool for researchers to manage their RNAi design projects. Its user-friendly interface makes it easy to navigate, and its step-by-step approach ensures that even beginners can use it with ease. Petunia's modular design allows for flexibility and scalability, making it suitable for businesses of all sizes. Additionally, Petunia integrates with other platforms via APIs, providing even more options for customization. Petunia also prioritizes data privacy, ensuring that all information is kept safe and secure.
Petunia offers three main differentiating factors:
- Performance: State of the art performance achieved by combining multiple datasets and robust machine learning models. This model can be fine-tuned on new data to tailor it to individual use cases.
- Explainability: Tools like feature importance and uncertainty quantification enable users to gain confidence in the models and enable informed decision-making.
- User Flow: Plug-and-play platform designed to make integration easy for researchers. Its stepwise, modular nature allows researchers to choose which functionalities to use, adjust parameters transparently and collect the outputs at each step, all in an intuitive user interface.
Performance
Petunia was developed with multiple publicly available datasets, namely the well-known Huesken dataset. To fully leverage the data, Loka experimented with several state of the art machine learning models, including sequence-based models and feature-based gradient boosting. The final model was obtained by removing the Huesken test data and using the remaining dataset for training and validating in a gene-fold-based cross validation setting, as to avoid data leakage. This model was ultimately evaluated on the Huesken test dataset, which it had never seen before. The table below shows the model test performance according to the Pearson Correlation Coefficient (PCC), which is the main evaluation metric in the literature. Results are compared against the two main literature standards, Biopredsi and i-Score. As can be seen, Petunia achieves state-of-the-art results.

For comparison purposes, we followed the same train/test split that is standard in the literature. We found this split to be unideal because it goes against the aforementioned gene-fold-based cross validation. Basically, having the same genes present on both train and test doesn’t adequately represent the real-world setting, because the deployed model will be mostly tested in unseen genes; this is especially critical if features are also extracted from the mRNA. Therefore, the literature standard evaluation setting suffers from data leakage. We will cover our team’s solutions to these and other challenges–such as other ranking metrics besides PCC–to better capture the real-world performance of our machine learning models. Stay tuned!
Finally, literature datasets are useful for evaluating the performance of a model, but true value is demonstrated only when the model is deployed in a real-world setting. For this purpose, it’s crucial to leverage the customer's data which is the best existing proxy of the production environment. That’s why the base model in Petunia is just a foundational model that can be further tailored to the customer's specific use cases. (At the end of this post we show a case study that shows how Petunia is used in the real world.)
Explainability
Stakes are high in the field of life sciences, and even the smallest error can have significant consequences. In critical applications like Petunia, model explainability is just as important as performance. Petunia offers the tools to explain and trust model results. And to better understand the explainability module, let’s consider an siRNA with the following guide strand:
AUGCAUUAGGUUGUUCACA
For this siRNA, Petunia predicted an efficacy of 20.8%. The true measured efficacy was 24.6%, so Petunia would have correctly avoided the testing of this siRNA. But what characteristics of this sequence most influenced the model’s prediction? Petunia’s explainability module gives us the answers.
The image below shows how specific nucleotides affect the predicted efficacy. For example, an A on the first position decreases the predicted efficacy by 6%. On the other hand, an A on the 19th position increases the predicted efficacy by 4%. The model has learned the fundamental rules that researchers have found over years of experimentation: an A as the first nucleotide is detrimental but as the last is beneficial.
Besides the existing known rules, Petunia has learned several more rules through the training process on vast amounts of data. This ability to go beyond rule-based approaches explains Petunia’s performance increase versus conventional methods.
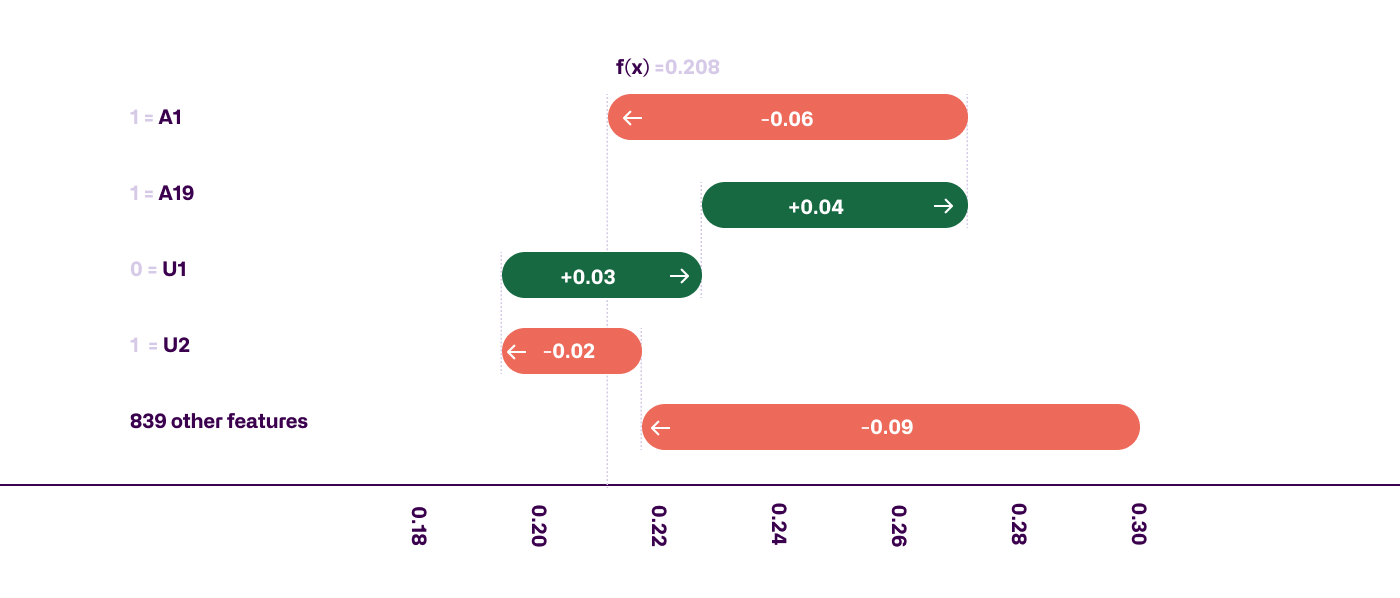
The ability to explain how each nucleotide affects the prediction is called “feature importance.” But Petunia offers several more features in its explainability module–namely the capability to understand how confident the model is of the prediction it is making. Has the model seen similar sequences in the training set? Or is it being tested out of distribution? All these explainability tools allow users to build trust in the model and are crucial to informed decision-making.
User Flow
A gene accession number is sufficient to start running the Petunia pipeline and for the generation of specific and potent siRNAs. The siRNA design process occurs transparently and sequentially, with parameters easily controlled and outputs of all processing steps effortlessly retrievable, from the mRNA target sequence space determination to the final ML-ranked siRNA sequences. Petunia’s modularity also allows the user to select the process step that best suits their interest (e.g. running the machine learning model inference for efficacy prediction on a siRNA shortlist uploaded by the user from its own internal pipeline), to mix and match different analysis within a project, and much more.
User Flow for Modules on Petunia
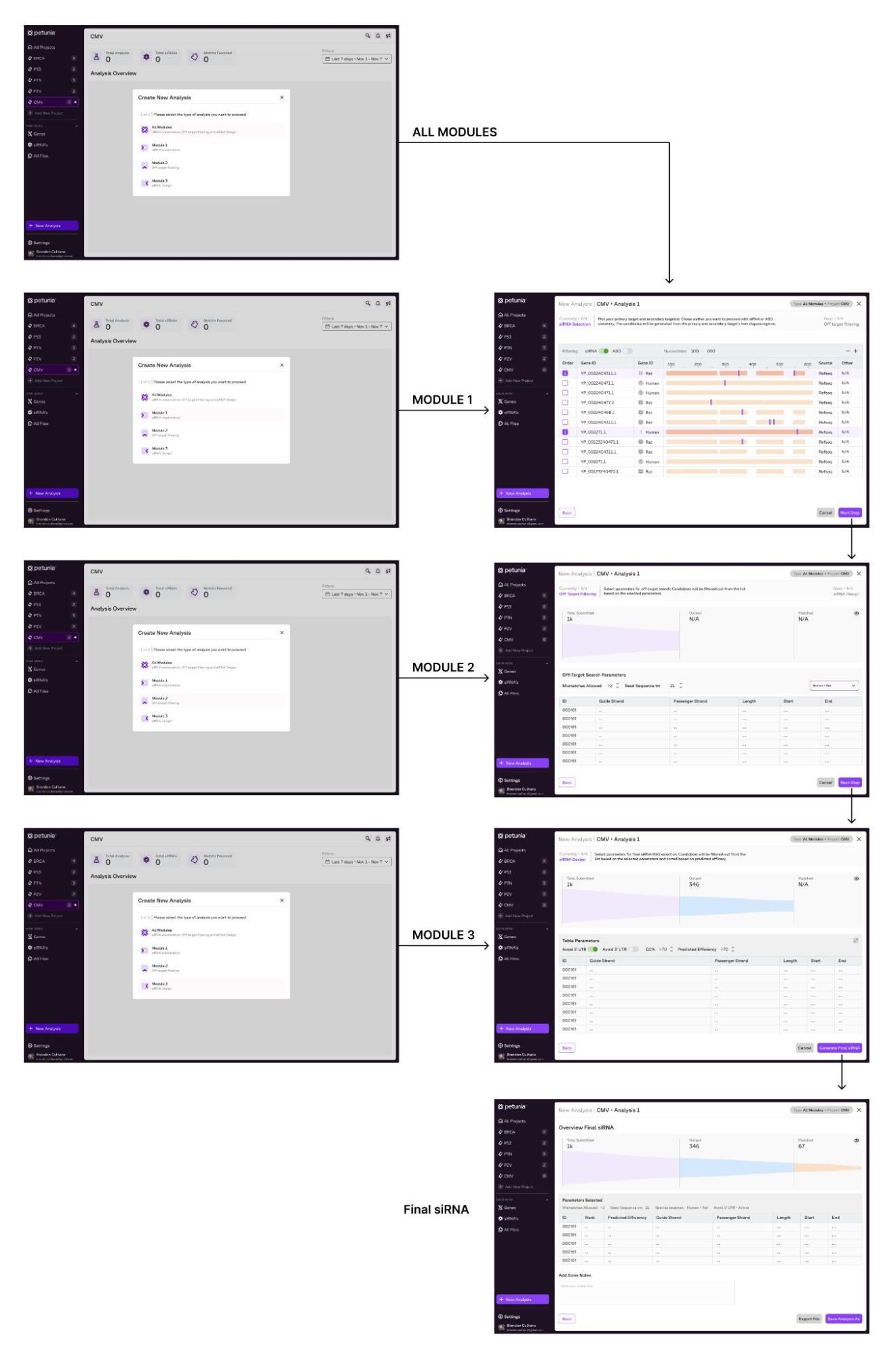
The Bottom Line
Petunia adds value to gene therapy businesses. Here’s how.
Assuming that no ML models were employed before and a target knockdown value of 70% or more of gene expression, we estimate that Petunia is able to discard around 30% of siRNAs before they’re tested in vitro, which leads to a cost-per-success decrease of around 9%, depending on the original rate of success of the siRNA design process.
Let’s consider the case of a lab that screened a big cohort of 1,000 siRNAs from their siRNA design pipeline, where each siRNA costs around $2,500 to produce and test. Their process had a ratio of approximately four successes per failure, identifying 786 effective siRNAs out of 1,000, which equates to a cost of ~$3,180 per success. Had they fed Petunia’s effectiveness prediction module (Module 3), ~30% of the siRNAs would be flagged–which equates to ~$750,000 worth of savings in would-be costs off the bat—while still identifying 589 successful candidates. The success rate would increase to six from four and the cost per success would decrease 8.5% to ~$2,911. This means that this lab could retrieve similar amounts of effective candidates while testing 30% less. This also means that in future projects, that same lab will spend $27,000 less for each 100 effective siRNAs it discovers.
Tomorrow’s Therapies Today
Incorporating advanced machine learning methods into RNAi design will accelerate the discovery of better candidates at a fraction of the cost. Many of the features and concepts used on ML-powered RNAi design are transferable between adjacent use cases such as antisense oligonucleotides (ASOs) and CRISPR-Cas9, meaning that advances in one case will ultimately improve the outcome of other DNA/RNA engineering tools.
As the field continues to advance technologically by incorporating new neural net architectures such as CNNs and LLMs, it also advances on the explainability power of these models. Explainability provides researchers a better understanding of the design process, and as a result, leads to the formulation of scientific hypotheses and the adjustment of in-house processes and methodologies. With Petunia, Loka provides a technologically powerful RNA design platform that delivers on explainability, usability and privacy.
Questions about Petunia? Ready for a demo? Reach out to Emily Kruger, Loka’s VP of Operations and Product, at emily@loka.com.